Scattering rates change across the Metal Insulator Transition¶
Explore the low energy expansion of the Matsubara self-energy. The zero frequency value being the scattering rate.
# Created Mon Mar 7 01:14:02 2016
# Author: Óscar Nájera
from __future__ import division, absolute_import, print_function
from math import log, ceil
import numpy as np
import matplotlib.pyplot as plt
import dmft.dimer as dimer
import dmft.common as gf
import dmft.ipt_imag as ipt
plt.matplotlib.rcParams.update({'axes.labelsize': 22,
'xtick.labelsize': 14, 'ytick.labelsize': 14,
'axes.titlesize': 22})
def loop_u_tp(u_range, tp_range, beta, seed='mott gap'):
"""Solves IPT dimer and return Im Sigma_AA, Re Simga_AB
returns list len(betarange) x 2 Sigma arrays
"""
tau, w_n = gf.tau_wn_setup(
dict(BETA=beta, N_MATSUBARA=max(2**ceil(log(4 * beta) / log(2)), 256)))
giw_d, giw_o = dimer.gf_met(w_n, 0., 0., 0.5, 0.)
if seed == 'I':
giw_d, giw_o = 1 / (1j * w_n - 4j / w_n), np.zeros_like(w_n) + 0j
sigma_iw = []
iterations = []
for tp, u_int in zip(tp_range, u_range):
giw_d, giw_o, loops = dimer.ipt_dmft_loop(
beta, u_int, tp, giw_d, giw_o, tau, w_n, 1e-3)
iterations.append(loops)
g0iw_d, g0iw_o = dimer.self_consistency(
1j * w_n, 1j * giw_d.imag, giw_o.real, 0., tp, 0.25)
siw_d, siw_o = ipt.dimer_sigma(u_int, tp, g0iw_d, g0iw_o, tau, w_n)
sigma_iw.append((siw_d.imag, siw_o.real))
print(np.array(iterations))
return sigma_iw
U_int = np.arange(.5, 4.5, 0.1)
sigmasM_Ur = loop_u_tp(U_int, .3 * np.ones_like(U_int), 200., 'M')
sigmasI_Ur = loop_u_tp(U_int[::-1], .3 * np.ones_like(U_int), 200., 'I')[::-1]
Out:
[187 17 27 31 22 36 31 31 35 40 44 39 38 42 41 40 39 38
37 40 35 34 32 30 28 24 22 19 16 13 12 4 4 4 4 4
4 4 4 3]
[ 5 4 4 4 4 4 4 4 4 4 4 4 4 4 5 5 5 6
6 6 8 10 14 119 41 46 42 43 43 44 40 45 40 41 31 27
36 22 32 26]
def plot_zero_w(function_array, iter_range, beta, entry, label_head, ax, dx):
"""Plot the zero frequency extrapolation of a function
Parameters
----------
function_array: real ndarray
contains the function (G, Sigma) to linearly fit over 2 first frequencies
iter_range: list floats
values of changing variable U or tp
berarange: real ndarray 1D, values of beta
entry: 0, 1 corresponds to diagonal or off-diagonal entry of function
label_head: string for label
ax, dx: matplotlib axis to plot in
"""
tau, w_n = gf.tau_wn_setup(dict(BETA=beta, N_MATSUBARA=20))
dat = []
for j, u in enumerate(iter_range):
dat.append(np.polyfit(w_n[:2], function_array[j][entry][:2], 1))
ax.plot(iter_range, -np.array(dat)[:, 1], label=label_head)
dx.plot(iter_range, -np.array(dat)[:, 0], label=label_head)
f, (si, ds) = plt.subplots(2, 2, sharex=True)
plot_zero_w(sigmasI_Ur, U_int, 100., 0, 'INS', si[0], ds[0])
plot_zero_w(sigmasM_Ur, U_int, 100., 0, 'MET', si[0], ds[0])
plot_zero_w(sigmasI_Ur, U_int, 100., 1, 'INS', si[1], ds[1])
plot_zero_w(sigmasM_Ur, U_int, 100., 1, 'MET', si[1], ds[1])
si[0].legend(loc=0, prop={'size': 8})
si[0].set_ylabel(r'-$\Im \Sigma_{AA}(w=0)$')
ds[0].set_ylabel(r'-$\Im d\Sigma_{AA}(w=0)$')
si[1].set_ylabel(r'-$\Re \Sigma_{AB}(w=0)$')
ds[1].set_ylabel(r'-$\Re d\Sigma_{AB}(w=0)$')
ds[0].set_xlabel('$U/D$')
ds[1].set_xlabel('$U/D$')
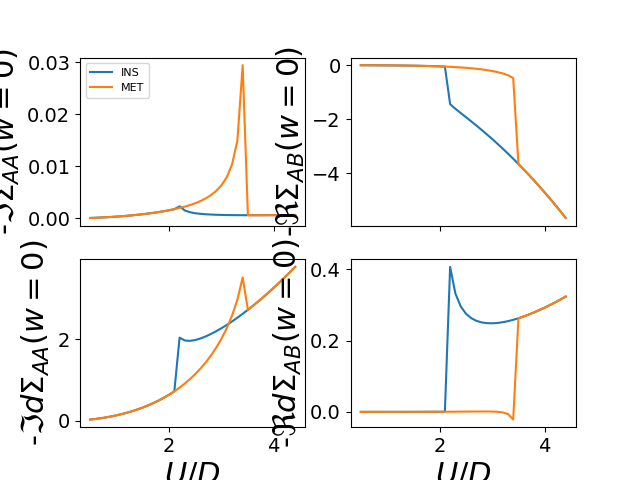
Total running time of the script: ( 0 minutes 5.798 seconds)